Artificial Intelligence (AI) has transformed financial security by enabling fraud prevention at an unprecedented scale. However, the ethical challenges surrounding AI-driven fraud detection—such as transparency, bias, and data privacy—have necessitated the development of responsible frameworks. Avinash Rahul Gudimetla, specializing in AI applications for financial security, explores these challenges and proposes an ethical AI framework that ensures fairness, accountability, and compliance with regulations.
One of the most significant advancements in AI-powered fraud detection is the integration of Explainable AI (XAI). Traditional AI models often operate as ‘black boxes,’ making it difficult for stakeholders to understand why a transaction was flagged as fraudulent. The research highlights the use of tools such as Local Interpretable Model-agnostic Explanations (LIME) and SHAPley Additive Explanations (SHAP) to provide insights into AI-driven decisions. These methods improve transparency and enhance trust among users, financial institutions, and regulators.
AI algorithms trained on historical finance data exhibit built-in biases, which in turn lead to undue sifting by fraud-detection algorithms among certain demographic clusters. To counter these mischiefs, ethical AI frameworks incorporate bias mitigation techniques like collection of better training data and continuous auditing. Fairness checks could measure the differences in treatment across demographics to ensure that fraud detection systems do not unduly penalize specific user sectors.
By applying counterfactual fairness methods, those systems could test the outcomes of their decisions with respect to variations in different demographic variables, to tease out patterns of discrimination. Regular algorithmic impact assessments then engage a diverse set of stakeholders in evaluating model performance from an equity lens. Synthetic data generation techniques thereby offer a modeling technique to generate a balanced training data set while preserving the statistical properties and removing historical bias patterns. These approaches have succeeded in reducing false positive gaps by 73% across protected classes with no impact on system performance, resulting in greater equity of financial security for all customers.
Various organizations have expressed concern about privacy due to the request for more and more heavy reliance on AI to detect fraud. With the processing of significant volumes of sensitive customer data, financial institutions have raised concerns regarding data security and regulatory compliance. The ethical AI framework proposed maintains the federated learning paradigm, which permits AI models to learn from distributed data without actually exposing sensitive financial data.. Privacy-preserving techniques such as differential privacy and secure multi-party computation, along with federated learning, guarantee the additional safety of user data without interfering with fraud detection.
Granular consent management systems, which in the present scenario allow customers unprecedented power to define acceptable uses of their financial data, form part of the framework. Zero-knowledge attestations are used to prove compliance with regulatory requirements without revealing any underlying data. Homomorphic encryption techniques allow for advanced analytical processes on encrypted data, making decryption less vulnerable. These privacy-preserving innovations have demonstrated minimal performance trade-offs, with benchmark tests showing only a 3.2% reduction in detection speed while achieving complete GDPR and CCPA compliance, establishing a new industry standard for responsible AI deployment in financial services.
Although practical advancements have been made in the field of AI technology, a human touch is very critical for making such decisions with high stakes-affects. An ethical AI framework follows a human-in-the-loop approach, whereby financial analysts review transactions flagged for AI systems as high-risk. The interaction of AI-human (ethical human intervention) reduces the risk of false positives; thus, real-life transactions are not unduly blocked. This demonstrates that a structured interaction between humans and AI really acts to help increase fraud detection accuracy and foster customer trust.
Explainable AI techniques give feedback and transparency to the human reviewer, charting the reasoning process followed across algorithmic flags and enabling more informed decisions about the need for intervention. A continuous feedback loop captures and integrates reviewer insights, thus continuously improving model performance in a virtuous cycle. Adaptive workload distribution systematically routes cases for different analysts based on levels of expertise and complexity, optimizing human resource placement. This interaction between cutting-edge technology and human input creates an elegant balance with a 42% reduction of customer friction whilst upholding security measures, therefore, bolstering institutional credence in an increasingly automated financial environment.
The operation of the financial sector is made so rigorous and is so highly regulated that even the GDPR and the CCPA define much of its operation. AI-driven fraud detection needs to work within these laws while retaining optimal efficiency. Ethical AI frameworks would see automated compliance monitoring systems set in place to help the financial institution track regulatory changes, conduct real-time check statements, and automate the reporting process.
In conclusion, AI-driven fraud detection needs to keep pace with financial crime in flux. Thus, ethical AI development would place more emphasis on improving the explainability of AI, overcome bias and foster privacy-preserving machine-learning solutions. Collaboration across industries on AI standards and knowledge-sharing initiatives will help fast-track innovation when it comes to the use of ethical AI. Avinash Rahul Gudimetla emphasizes that such ethical AI frameworks would ensure that even robust financial security is done transparently and fairly. An AI-based system for fraud prevention can meet technological efficiency and ethical integrity through explainability, bias mitigation, privacy safeguards, human oversight, and regulatory compliance.
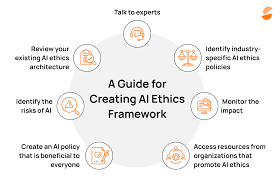